Pain therapy is one of the most important areas of health care with 12–15 million patients with chronic pain, including 5 million with severe pain, in Germany alone. Worldwide, more than 100 million patients take opioid-based medications, despite the significant side effects, including death. These numbers are rising and can lead to a societal crisis, as the current “opioid epidemic” in the USA with several hundred thou-sand deaths shows [4].
Our goal was to develop new drugs that produce the desired effect (relief of severe pain) but not the undesirable side effects (e.g. respiratory distress, addiction, cardiac arrest). When developing drugs, we try to find a small molecule, called a “ligand” that can bind to a specific target molecule on the surface of our cells and, by binding, induce the desired effect, in this case pain relief. The problem is that most ligand molecules bind not only where the effect is needed (in diseased, e.g. inflamed, tissue), but also where they cause side effects (e.g. in healthy tissue in the brain). Diseased and healthy tissue differ by the chemical environment of the affected cells in our body. Therefore, the idea was to find ligands that only bind to the cell surface where needed – in the chemical environment characteristic of the disease. This represents a new paradigm of drug design that was proposed only a few years ago jointly by ZIB researchers and physicians from the Charité University Hospital [11].
This team succeeded in finding a ligand molecule that actually binds only in inflamed tissue and not in healthy tissue. The development was achieved without laboratory or animal experiments, but by using ZIB’s supercomputer [10]. To do this, we had to solve a serious problem: The calculations needed to understand whether a ligand binds better in inflamed than in healthy tissue require an enormous amount of computing time on the supercomputer; the power consumption alone would cost millions of euros and produce thousands of tons of CO2. Together with other mathematicians from the MATH+ Cluster of Excellence, we overcame this obstacle. We succeeded in developing new mathematical techniques to perform the calculations in an intelligent and very efficient way, reducing the computing time to days and the electricity consumption and CO2 production to less than a thousandth [1, 6–8].
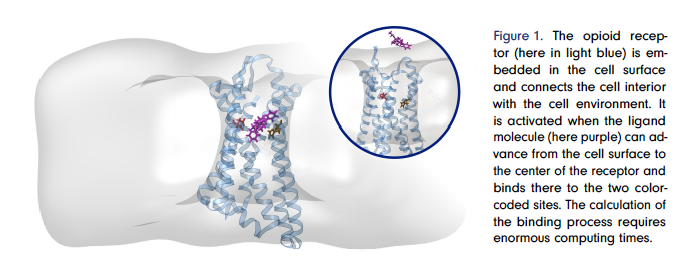
The new drug candidate has been patented [2, 12] and medical experiments have shown that it does indeed relieve severe pain and also works solely in inflamed tissue as calculated [5, 13]. Clinical trials are currently being conducted in collaboration with Queen’s University Canada to see if it really does have no or very mild side effects.
Inspired by this success, we are now working to use the new mathematical techniques and the novel drug design paradigm to develop other non-addictive drugs. Not only for pain relief, but also for other serious diseases such as cancer, COVID-19 [14] or cardiovascular disease. To this end, our team, in collaboration with Harvard University, has developed a highly automated software platform that is able to search for new drugs in huge libraries of billions of candidate molecules on supercomputers [3]. With success: new drug candidates have already been found and are currently being chemically produced and tested in detail [2].
In the future, we want to go much further than this approach. We are working on a way to use artificial intelligence to suggest new chemical substances with “drug-like” structures [9]. In this way, we can find substances that are completely new and have never been considered as drug candidates. Initial results are very promising.
References:
[1] L. Donati and M. Weber. Assessing transition rates as functions of environmental variables. J. Chem. Phys. 157.22 (Dec. 2022), 224103. DOI: 10.1063/5. 0109555.
[2] Drug candidates as pareto optima in chemical space. European patent application No. EP24162096.2. 2024.
[3] C. Gorgulla, A. Boeszoermenyi, Z.-F. Wang, P. D. Fischer, P. W. Coote, K. M. P. Das, Y. S. Malets, D. S. Radchenko, Y. S. Moroz, D. A. Scott, K. Fackeldey, M. Hoffmann, I. Iavniuk, G. Wagner, and H. Arthanari. An open-source drug discovery platform enables ultra-large virtual screens. Nature 580.5 (2020), 663–668. DOI: 10.1038/s41586-020-2117-z.
[4] M. S. Jalali, M. Botticelli, R. C. Hwang, H. K. Koh, and R. K. McHugh. The opioid crisis: need for systems science research. Health Research Policy and Systems 18(88) (2020). DOI: 10.1186/s12961-020-00598-6.
[5] J. Möller, A. Isbilir, T. Sungkaworn, B. Osberg, C. Karathanasis, V. Sunkara, E. O. Grushevsky, A. Bock, P. Annibale, M. Heilemann, C. Schütte, and M. J. Lohse. Single molecule mu-opioid receptor membrane-dynamics reveal agonist-specific dimer formation with super-resolved precision. Nature Chemical Biology 16 (2020), 946–954. DOI: 10.1038/s41589-020-0566-1.
[6] R. J. Rabben, S. Ray, and M. Weber. ISOKANN: in-variant subspaces of Koopman operators learned by a neural network. The Journal of Chemical Physics 153.11 (2020), 114109. DOI: 10.1063/5.0015132.
[7] S. Ray, V. Sunkara, C. Schütte, and M. Weber. How to calculate pH-dependent binding rates for receptor-ligand systems based on thermodynamic simulations with different binding motifs. Molecular Simulation 46.18 (2020), 1443–1452. DOI: 10.1080/08927022. 2020.1839660.
[8] C. Schütte, S. Klus, and C. Hartmann. Overcoming the timescale barrier in molecular dynamics: transfer operators, variational principles, and machine learning. Acta Numerica 32 (2023), 517–673. DOI: 10.1017/S0962492923000016.
[9] C. Secker, K. Fackeldey, M. Weber, S. Ray, C. Gorgulla, and C. Schütte. Novel multi-objective affinity approach allows to identify pH-specific 𝜇-opioid receptor agonists. Journal of Cheminformatics 15 (2023). DOI: 10.1186/s13321-023-00746-4.
[10] V. Spahn, G. D. Vecchio, D. Labuz, A. Rodriguez-Gaztelumendi, N. Massaly, J. Temp, V. Durmaz, P. Sabri, M. Reidelbach, H. Machelska, M. Weber, and C. Stein. A nontoxic pain killer designed by modeling of pathological receptor conformations. Science 355.6328 (2017), 966–969. DOI: 10.1126/science
[11] C. Stein, M. Weber, O. Scharkoi, and P. Deuflhard. Method and system for indentifying compounds that bind and preferably activate a target opioid receptor in a pH-dependent manner. EP2613277.
[12] C. Stein, C. Zöllner, M. Weber, and O. Scharkoi. Fentanyl derivatives as pH-dependent opioid receptor agonists. EP2559685.
[13] A. Thies, V. Sunkara, S. Ray, H. Wulkow, M. Ö. Celik, F. Yergöz, C. Schütte, C. Stein, M. Weber, and S. Winkelmann. Modelling altered signalling of G-protein coupled receptors in inflamed environment to advance drug design. Scientific Reports 13.607 (2023). DOI: 10.1038/s41598-023-27699-w.
[14] P. Trepte, C. Secker, S. Kostova, S. B. Maseko, S. G. Choi, J. Blavier, I. Minia, E. S. Ramos, P. Casson-net, S. Golusik, M. Zenkner, S. Beetz, M. J. Liebich, N. Scharek, A. Schütz, M. Sperling, M. Lisurek, Y. Wang, K. Spirohn, T. Hao, M. A. Calderwood, D. E. Hill, M. Landthaler, J. Olivet, J.-C. Twizere, M. Vidal, and E. E. Wanker. AI-guided pipeline for protein-protein interaction drug discovery identifies a SARS-CoV-2 inhibitor. Molecular Systems Biology (2023). DOI: 10.1101/2023.06.14.544560.