This research is carried out in the framework of MATHEON supported by Einstein Foundation Berlin.
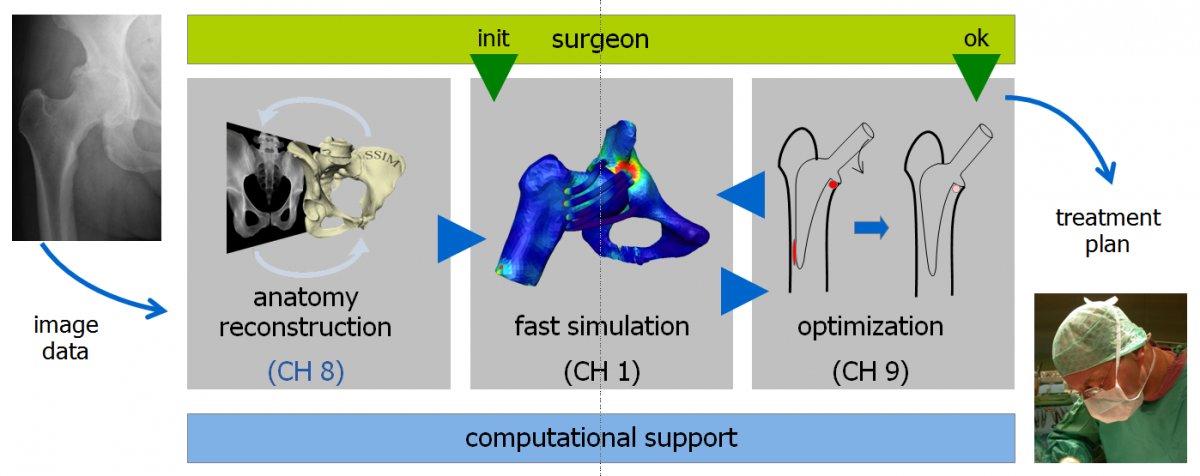
Anatomical models have become an indispensable tool in preoperative surgery planning and outcome assessment, e.g., in knee or hip replacement as considered in the companion projects CH1 (Reduced basis methods in orthopedic hip surgery planning) and CH9 (Adaptive Algorithms for Optimization of Hip Implant Positioning).
Modern medicine relies heavily on X-ray imaging. The ionizing radiation, however, damages biological tissue; at least 1.5% of cancer cases are attributed to exposure to X-rays in medical examinations. Dose reduction therefore remains an important goal. Since the dose of 1 CT corresponds to the dose of 250–500 radiographs, substitution of CT by 2D radiographs is an important subgoal.
Computer-based treatment planning is based on 3D anatomical models. In case of bony structures such models are computed from CTs. In the parallel project 3D from X-ray it was shown that such 3D models can be computed also from 2D radiographs, if strong priors (statistical shape and intensity models) are used. The goal of this project is to further advance these methods such that a requested reconstruction accuracy with minimal radiation dose is achieved. For this we will develop
- a multilevel/multiresolution methods for both the shape model coefficients and image data,
- optimal design of experiment methods for selecting best view angles and X-ray intensities and
- fast design optimization algorithms to optimize individual imaging plans on the fly.
Method
A 3D reconstruction from few 2D radiographs is an ill-posed inverse problem. For that fitting a detailed articulated statistical shape and intensity model (ASSIM) to planar radiographs is a promising method. The procedure of generating radiographs is simulated by a virtual X-ray projection of an anatomical bone model as the forward process. By formulating the 3D from X-ray reconstruction as a Bayesian inverse problem, the effect of the radiography noise induced uncertainty can be captured and analyzed. This formulation leads to a posterior distribution consisting the ASSIM prior and a likelihood measuring the mismatch between the real and virtual X-ray images. We consider the Maximum A Posteriori (MAP) as a point estimation together with the posterior covariance matrix for the uncertainty quantification. This information are essential and necessary for further investigations such as the Design of Experiments (DoE) method for individual imaging plans.
Point Estimation
In contrast to the prior the likelihood distribution, including the nonlinear forward process, is not Gaussian. Therefore finding the MAP leads to a nonlinear optimization problem solved by gradient-based optimization strategies. For that analytical gradient information of the virtual X-ray projection are used with an example shown in Fig. 1.
Fig. 1. Virtual X-ray projection of mean hip (left) and its derivative w.r.t. a shape parameter (right).
For a correct gradient computation the image resolution is highly relevant in the vicinity of contours to avoid aliasing artefacts. For that an adaptive choice of local supersampling will therefore be considered. In Fig. 2. the difference between normal and supersampled low resolution derivative image is clarified.
Fig. 2. Left: Low resolution of virtual X-ray projection derivative. Right: Supersampling of the same derivative followed by downsampling to the same resolution as left. The information is more detailed and a better approximation to the real physical process in contrast to the left image.
Uncertainty Quantification
In view of optimal design of experiments, we aim at a fast local uncertainty quantification based on second order Taylor approximation around the MAP based on the hypothesis that the posterior is approximately Gaussian. This property could be verified by sampling the posterior with an adaptive Metropolis algorithm. By a first order Taylor replacement of the virtual X-ray projection in the likelihood the posterior distribution becomes Gaussian and an approximation of the posterior covariance matrix can be calculated very fast. But comparing the sample covariance from the Metropolis algorithm with the approximated covariance is often unsatisfactory, with a variance too small by a factor up to three in practical situations (Fig. 3).
Fig. 3. Blue: Histogram of samples generated from adaptive Metropolis algorithm for one shape parameter. Red: Best Gaussian distribution fit to histogram. Black: Gaussian distribution from Taylor approximation. The real variance can be captured well by Gaussian but is underestimated by the approximation.
The virtual X-ray projection depends highly nonlinear on the ASSIM-depending deformation. The reason is, that shape parameter variations leading to translation of sharp contours in the projection image which give rise to a Laplacian distribution as posterior. Image smoothing leads to better Taylor approximation of the posterior on the one hand but increases the posterior variance on the other which at the sime time provides an upper bound for the uncertainty.
Design of Experiments
An accurate covariance estimation of the posterior can also be used for different uncertainty quantification kinds of the MAP, a possible geometric variation caused by uncertainty is shown in Fig. 4. left. Finding best view angle and radiation dose for the X-ray recording setup for minimizing patient specific radiation exposure under requested reconstruction accuracy therefore leads to an optimal design of experiment problem (Fig. 4. right).
Fig. 4. Normal displacement uncertainty computed from the ASSIM parameter covariance. Right: Uncertainty for single XR projection depending on the view angle.
Individual Imaging Plan
If the requested reconstruction accuracy can not be reached with a single XR projection additional information of the patient specific bone anatomy for further uncertainty reduction is necessary. For that, at a given reconstruction state find the best uncertainty reducing recording setup to receive the new radiography and use the posterior as new prior for a further reconstruction process. Since the uncertainty can not rise under additional information,, this iterative procedure can be repeated until the requested reconstruction accuracy is reached.