Deep learning methods excel in various do-mains, yielding substantial economic benefits and practical implications. From powering self-driving cars and enabling protein folding solutions in drug development to driving recommendation algorithms in major social networks, these methods demonstrate remarkable versatility. Large Language Models like ChatGPT are notable examples, processing vast amounts of data with increasing efficiency. However, their widespread application brings to light a critical issue: the opaque, “black-box” nature of these models. This lack of transparency, particularly in parameter-dense and highly complex neural networks, poses significant challenges in sensitive areas such as medical diagnosis and autonomous driving, where clear and understandable reasoning is crucial for trust and reliability.
To more clearly illustrate the point let us consider the Clever Hans phenomenon, which pro-vides a fascinating glimpse into the complexities of interpreting animal behavior and, by extension, the challenges in understanding how artificial intelligence systems make decisions. Hans was a horse that lived in Germany at the turn of the 20th century, and he became famous for his supposed ability to perform arithmetic and other intellectual tasks. His owner, Wilhelm von Osten, a phrenologist and retired math teacher from Berlin, believed Hans could understand German and respond to questions by tapping his hoof. For instance, if asked a simple math question, Hans would tap his hoof the correct number of times to indicate the answer.
It turned out that Hans was not actually per-forming calculations or understanding language. Instead, the horse was incredibly sensitive to subtle, involuntary cues in the body language of his trainer and the audience around him. For example, when the correct number of taps was reached, the trainer’s posture and expressions would —however slightly— change indicating to Hans that he should stop tapping.
This discovery highlighted that it was not the intellectual prowess of the horse at play but a nuanced form of communication based on visual cues that the human observers were giving off without realizing it; a side channel. In the context of AI, the Clever Hans phenomenon underscores the necessity for explainability. Just as Hans’ apparent intelligence was actually a reflection of human cues, AI systems might “learn” from data in ways that reflect underlying biases or patterns invisible to their developers. Without a clear understanding of how and why AI algorithms arrive at their decisions, we risk misinterpreting their capabilities and relying on their judgments in situations where they might not be valid or fair.
At ZIB, we have been working on Explainable Artificial Intelligence (XAI) in order to make neu-ral networks transparent and understandable, with the goal of ensuring that these AI systems are not only effective but also adhere to ethical and social standards. In the future we aim to extend our research to both text and learned feature spaces with the aim of deepening the understanding of interpretability across different modalities and data types. This is particularly important in the evolving AI regulatory landscape, such as the European Union’s AI law, where interpretability of AI systems plays a crucial role.
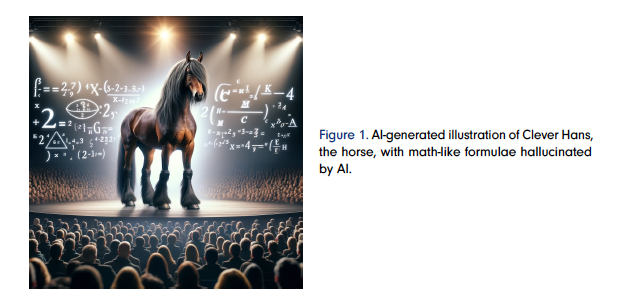